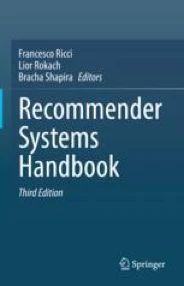
Abstract
This chapter studies state-of-the-art research related to multimedia recommender systems (MMRS), focusing on methods that integrate multimedia content as side information to various recommendation models. The multimedia features are then used by an MMRS to recommend either (1) media items from which the features were derived, or (2) non-media items utilizing the features obtained from a proxy multimedia representation of the item (e.g., images of clothes). We first outline the key considerations and challenges that must be taken into account while developing an MMRS. We then discuss the most popular multimedia content processing approaches to produce item representations that may be utilized as side information in an MMRS. Finally, we discuss recent state-of-the-art MMRS algorithms, which we classify and present according to classical hybrid models (e.g., VBPR), neural approaches, and graph-based approaches. Throughout this work, we mentioned several use-cases of MMRSs in the recommender systems research across several domains or products types such as food, fashion, music, videos, and so forth. We hope this chapter provides fresh insights into the nexus of multimedia and recommender systems, which could be exploited to broaden the frontier in the field.
Citation
Yashar Deldjoo,
Markus
Schedl,
Balazs Hidasi,
Yinwei Wei,
Xiangnan He
Multimedia Recommender Systems: Algorithms and Challenges
Recommender Systems Handbook (3rd edition),
973--1014, doi:10.1007/978-1-0716-2197-4_25, 2022.
BibTeX
@incollection{Deldjoo2022Multimedia, title = {Multimedia Recommender Systems: Algorithms and Challenges}, author = {Deldjoo, Yashar and Schedl, Markus and Hidasi, Balazs and Wei, Yinwei and He, Xiangnan}, booktitle = {Recommender Systems Handbook (3rd edition)}, editor = {Ricci, Francesco and Rokach, Lior and Shapira, Bracha}, publisher = {Springer}, address = {New York, NY}, doi = {10.1007/978-1-0716-2197-4_25}, url = {https://doi.org/10.1007/978-1-0716-2197-4_25}, pages = {973--1014}, year = {2022} }