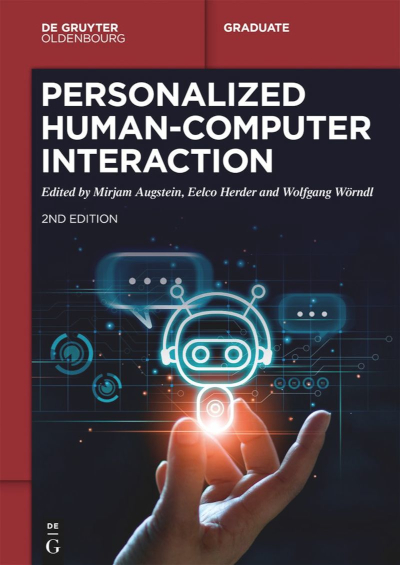
Abstract
Information access systems, such as search engines and recommender systems, affect many day-to-day decisions in modern societies by preselecting and ranking content users are exposed to on the web (e. g., products, music, movies or job advertisements). While they have undoubtedly improved users’ opportunities to find useful and relevant digital content, these systems and their underlying algorithms often exhibit several undesirable characteristics. Among them, harmful biases play a significant role and may even result in unfair or discriminating behavior of such systems. In this chapter, we give an introduction to the different kinds and sources of biases from various perspectives as well as their relation to algorithmic fairness considerations. We also review common computational metrics that formalize some of these biases. Subsequently, the major strategies to mitigate harmful biases are discussed and each is illustrated by presenting concrete state-of-the-art approaches from scientific literature. Finally, we round off by identifying open challenges in research on fair information access systems.
Citation
Markus
Schedl,
Elisabeth Lex
Fairness of information access system
Personalized Human-Computer Interaction,
59--78, doi:10.1515/9783110988567-003, 2023.
BibTeX
@incollection{Schedl2023Fairness, title = {Fairness of information access system}, author = {Schedl, Markus and Lex, Elisabeth}, booktitle = {Personalized Human-Computer Interaction}, editor = {Augstein, Mirjam and Herder, Eelco and Wörndl, Wolfgang}, publisher = {De Gruyter Oldenbourg}, address = {Berlin, Boston}, doi = {10.1515/9783110988567-003}, url = {https://doi.org/10.1515/9783110988567-003}, isbn = {9783110988567}, pages = {59--78}, year = {2023} }