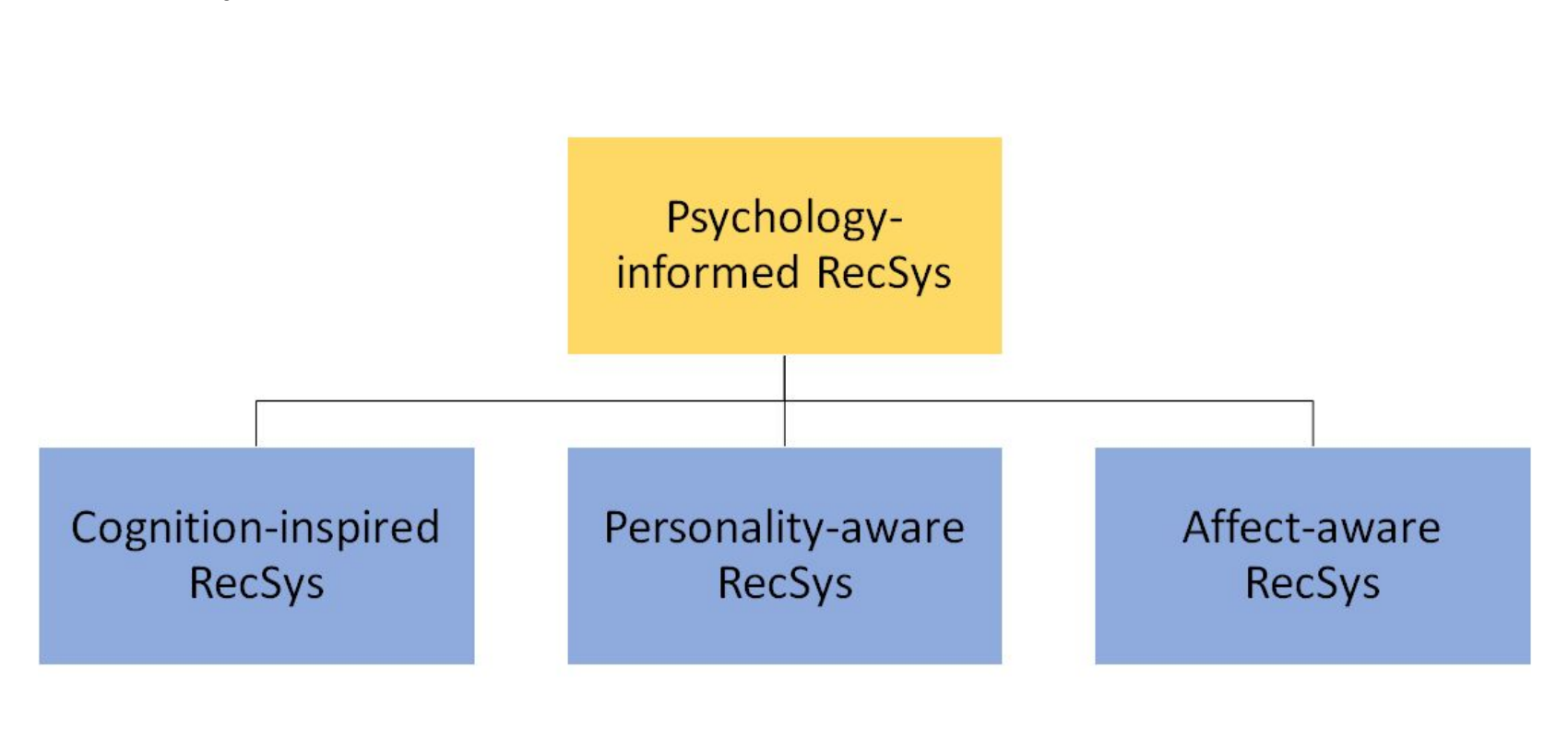
Abstract
Personalized recommender systems have become indispensable in today’s online world. Most of today’s recommendation algorithms are data-driven and based on behavioral data. While such systems can produce useful recommendations, they are often uninterpretable, black-box models, which do not incorporate the underlying cognitive reasons for user behavior in the algorithms’ design. The aim of this survey is to present a thorough review of the state of the art of recommender systems that leverage psychological constructs and theories to model and predict user behavior and improve the recommendation process. We call such systems psychology-informed recommender systems. The survey identifies three categories of psychology-informed recommender systems: cognition-inspired, personality-aware, and affectaware recommender systems. Moreover, for each category, we highlight domains, in which psychological theory plays a key role and is therefore considered in the recommendation process. As recommender systems are fundamental tools to support human decision making, we also discuss selected decision-psychological phenomena that impact the interaction between a user and a recommender. Besides, we discuss related work that investigates the evaluation of recommender systems from the user perspective and highlight user-centric evaluation frameworks. We discuss potential research tasks for future work at the end of this survey.
Citation
Elisabeth Lex,
Dominik Kowald,
Paul Seitlinger,
Thi Ngoc Trang Tran,
Alexander Felfernig,
Markus
Schedl
Psychology-Informed Recommender Systems
Foundations and Trends in Information Retrieval,
15(2):
134-242, doi:10.1561/1500000090, 2021.
BibTeX
@article{Lex2021PsychInformed, title = {Psychology-Informed Recommender Systems}, author = {Lex, Elisabeth and Kowald, Dominik and Seitlinger, Paul and Tran, Thi Ngoc Trang and Felfernig, Alexander and Schedl, Markus}, journal = {Foundations and Trends in Information Retrieval}, publisher = {Now Publishers Inc.}, address = {Hanover, MA, USA}, doi = {10.1561/1500000090}, url = {https://doi.org/10.1561/1500000090}, volume = {15}, number = {2}, pages = {134-242}, month = {jul}, year = {2021} }