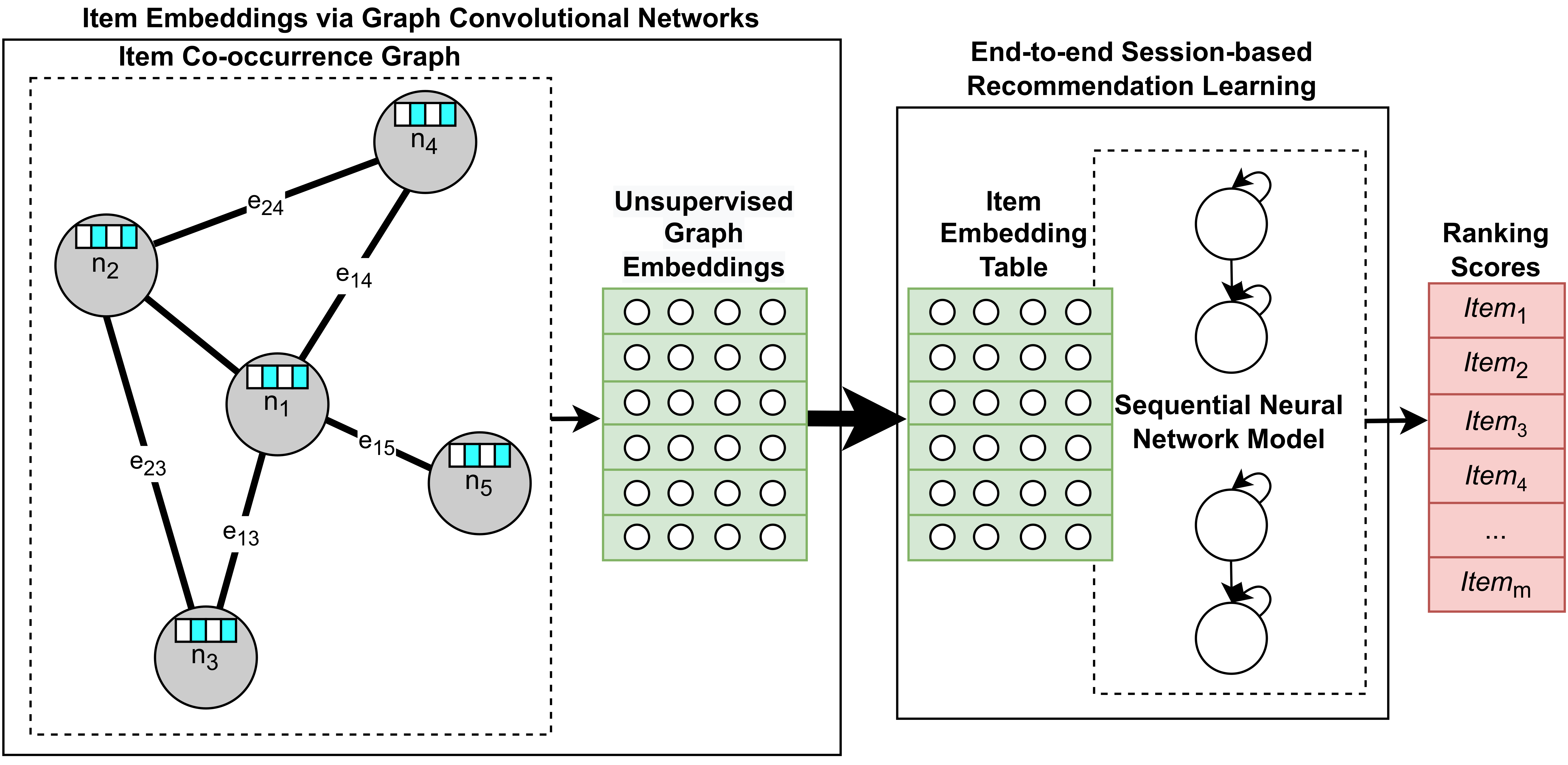
Abstract
In session-based recommender systems, predictions are based on the user’s preceding behavior in the session. State-of-the-art sequential recommendation algorithms either use graph neural networks to model sessions in a graph or leverage the similarity of sessions by exploiting item features. In this paper, we combine these two approaches and propose a novel method, Graph Convolutional Network Extension (GCNext), which incorporates item features directly into the graph representation via graph convolutional networks. GCNext creates a feature-rich item co-occurrence graph and learns the corresponding item embeddings in an unsupervised manner. We show on three datasets that integrating GCNext into sequential recommendation algorithms significantly boosts the performance of nearest–neighbor methods as well as neural network models. Our flexible extension is easy to incorporate in state-of-the-art methods and increases the MRR@20 by up to 12.79%.
Citation
Andreas Peintner,
Marta
Moscati,
Emilia
Parada-Cabaleiro,
Markus
Schedl,
Eva Zangerle
Unsupervised Graph Embeddings for Session-based Recommendation with Item Features
CARS Workshop on Context-Aware Recommender Systems (RecSys), 2022.
BibTeX
@article{AndreasPeintner2022GCNext, title = {Unsupervised Graph Embeddings for Session-based Recommendation with Item Features}, author = {Andreas Peintner and Moscati, Marta and Parada-Cabaleiro, Emilia and Schedl, Markus and Eva Zangerle}, booktitle = {CARS Workshop on Context-Aware Recommender Systems (RecSys)}, publisher = {Association for Computing Machinery}, address = {New York, NY, USA}, location = {Seattle, WA, USA}, year = {2022} }