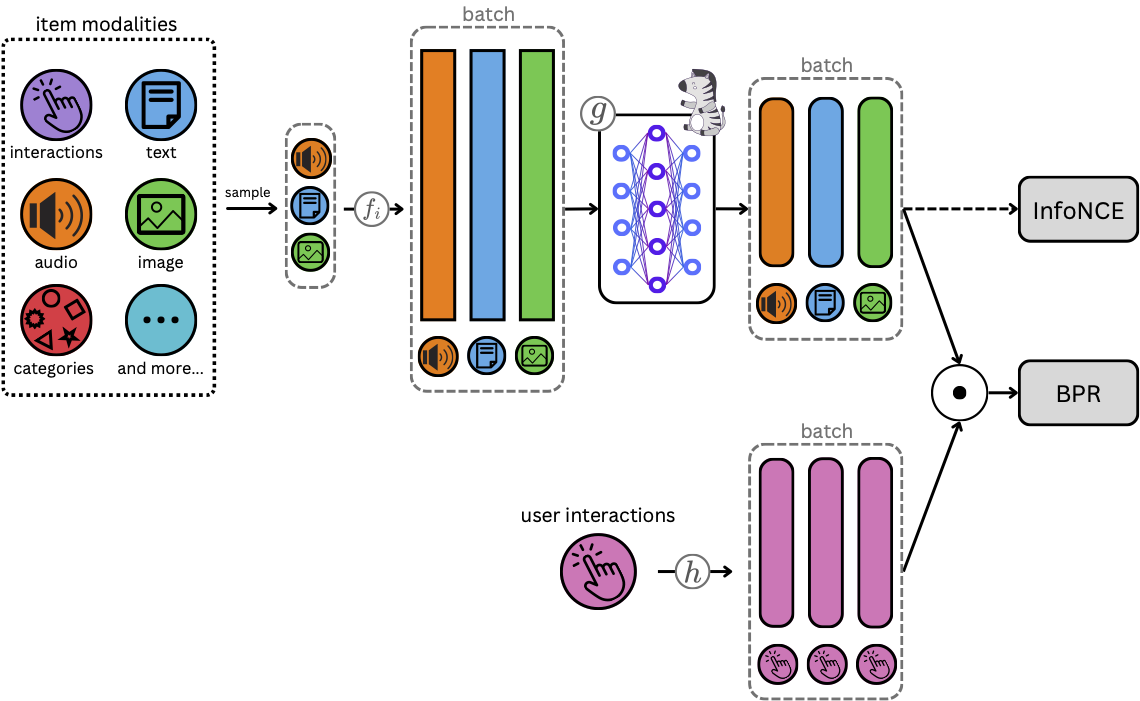
Abstract
Most recommender systems adopt collaborative filtering (CF) and provide recommendations based on past collective interactions. Therefore, the performance of CF algorithms degrades when few or no interactions are available, a scenario referred to as cold-start. To address this issue, previous work relies on models leveraging both collaborative data and side information on the users or items. Similar to multimodal learning, these models aim at combining collaborative and content representations in a shared embedding space. In this work we propose a novel technique for multimodal recommendation, relying on a multimodal Single-Branch embedding network for Recommendation (SiBraR). Leveraging weight-sharing, SiBraR encodes interaction data as well as multimodal side information using the same single-branch embedding network on different modalities. This makes SiBraR effective in scenarios of missing modality, including cold start. Our extensive experiments on large-scale recommendation datasets from three different recommendation domains (music, movie, and e-commerce) and providing multimodal content information (audio, text, image, labels, and interactions) show that SiBraR significantly outperforms CF as well as state-of-the-art content-based RSs in cold-start scenarios, and is competitive in warm scenarios. We show that SiBraR's recommendations are accurate in missing modality scenarios, and that the model is able to map different modalities to the same region of the shared embedding space, hence reducing the modality gap.
Citation
Christian
Ganhör,
Marta
Moscati,
Anna
Hausberger,
Shah
Nawaz,
Markus
Schedl
A Multimodal Single-Branch Embedding Network for Recommendation in Cold-Start and Missing Modality Scenarios
Proceedings of the 18th ACM Conference on Recommender Systems (RecSys),
380–390, doi:10.1145/3640457.3688138, 2024.
BibTeX
@inproceedings{ganhoer_moscati2024sibrar, title = {A Multimodal Single-Branch Embedding Network for Recommendation in Cold-Start and Missing Modality Scenarios}, author = {Ganhör, Christian and Moscati, Marta and Hausberger, Anna and Nawaz, Shah and Schedl, Markus}, booktitle = {Proceedings of the 18th ACM Conference on Recommender Systems (RecSys)}, publisher = {Association for Computing Machinery}, address = {New York, NY, USA}, url = {https://doi.org/10.1145/3640457.3688138}, doi = {10.1145/3640457.3688138}, pages = {380–390}, location = {Bari, Italy}, year = {2024} }