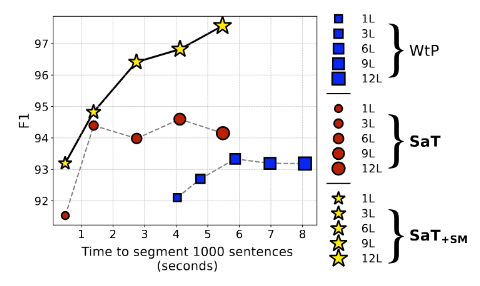
Abstract
Segmenting text into sentences plays an earlyand crucial role in many NLP systems. This iscommonly achieved by using rule-based or sta-tistical methods relying on lexical features suchas punctuation. Although some recent worksno longer exclusively rely on punctuation, wefind that no prior method achieves all of (i) ro-bustness to missing punctuation, (ii) effectiveadaptability to new domains, and (iii) high effi-ciency. We introduce a new model — Segmentany Text (SAT) — to solve this problem. To en-hance robustness, we propose a new pretrainingscheme that ensures less reliance on punctua-tion. To address adaptability, we introduce anextra stage of parameter-efficient fine-tuning,establishing state-of-the-art performance in dis-tinct domains such as verses from lyrics andlegal documents. Along the way, we introducearchitectural modifications that result in a three-fold gain in speed over the previous state of theart and solve spurious reliance on context farin the future. Finally, we introduce a variant ofour model with fine-tuning on a diverse, mul-tilingual mixture of sentence-segmented data,acting as a drop-in replacement and enhance-ment for existing segmentation tools. Overall,our contributions provide a universal approachfor segmenting any text. Our method outper-forms all baselines — including strong LLMs— across 8 corpora spanning diverse domainsand languages, especially in practically relevantsituations where text is poorly formatted.
Citation
Markus Frohmann,
Igor Sterner,
Ivan Vulic,
Benjamin Minixhofer,
Markus
Schedl
Segment Any Text: A Universal Approach for Robust, Efficient and Adaptable Sentence Segmentation
Proceedings of the 2024 Conference on Empirical Methods in Natural Language Processing (EMNLP),
11908–11941, doi:10.18653/v1/2024.emnlp-main.665, 2024.
BibTeX
@inproceedings{MarkusFrohmann2024acl_segment, title = {Segment Any Text: A Universal Approach for Robust, Efficient and Adaptable Sentence Segmentation}, author = {Markus Frohmann and Igor Sterner and Ivan Vulic and Benjamin Minixhofer and Schedl, Markus}, booktitle = {Proceedings of the 2024 Conference on Empirical Methods in Natural Language Processing (EMNLP)}, editor = {Yaser Al-Onaizan, Mohit Bansal, Yun-Nung Chen}, publisher = {Association for Computing Machinery}, address = {Miami, Florida, USA}, doi = {10.18653/v1/2024.emnlp-main.665}, url = {https://aclanthology.org/2024.emnlp-main/}, pages = {11908–11941}, month = {November}, year = {2024} }