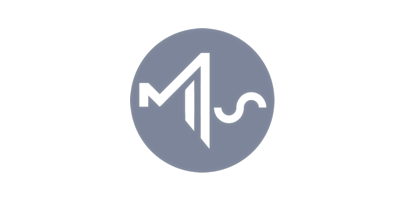
Abstract
Language models frequently inherit societalbiases from their training data. Numerous tech-niques have been proposed to mitigate thesebiases during both the pre-training and fine-tuning stages. However, fine-tuning a pre-trained debiased language model on a down-stream task can reintroduce biases into themodel. Additionally, existing debiasing meth-ods for downstream tasks either (i) require la-bels of protected attributes (e.g., age, race, orpolitical views) that are often not available or(ii) rely on indicators of bias, which restrictstheir applicability to gender debiasing sincethey rely on gender-specific words. To addressthis, we introduce a novel debiasing regulariza-tion technique based on the class-wise varianceof embeddings. Crucially, our method doesnot require attribute labels and targets any at-tribute, thus addressing the shortcomings ofexisting debiasing methods. Our experimentson encoder language models and three datasetsdemonstrate that our method outperforms ex-isting strong debiasing baselines that rely ontarget attribute labels while maintaining perfor-mance on the target task.
Citation
Shahed
Masoudian,
Markus Frohmann,
Navid Rekabsaz,
Markus
Schedl
Unlabeled Debiasing in Downstream Tasks via Class-wise Low Variance Regularization
Proceedings of the 2024 Conference on Empirical Methods in Natural Language Processing (EMNLP),
10932-10938, doi:10.18653/v1/2024.emnlp-main.612, 2024.
BibTeX
@masoudian-etal-2024-unlabeled{Masoudian2024acl_lowvariancereg, title = {Unlabeled Debiasing in Downstream Tasks via Class-wise Low Variance Regularization}, author = {Masoudian, Shahed and Markus Frohmann and Navid Rekabsaz and Schedl, Markus}, booktitle = {Proceedings of the 2024 Conference on Empirical Methods in Natural Language Processing (EMNLP)}, editor = {Yaser Al-Onaizan, Mohit Bansal, Yun-Nung Chen}, publisher = {Association for Computing Machinery}, address = {Miami, Florida, USA}, doi = {10.18653/v1/2024.emnlp-main.612}, url = {https://aclanthology.org/2024.emnlp-main/}, pages = {10932-10938}, month = {November}, year = {2024} }