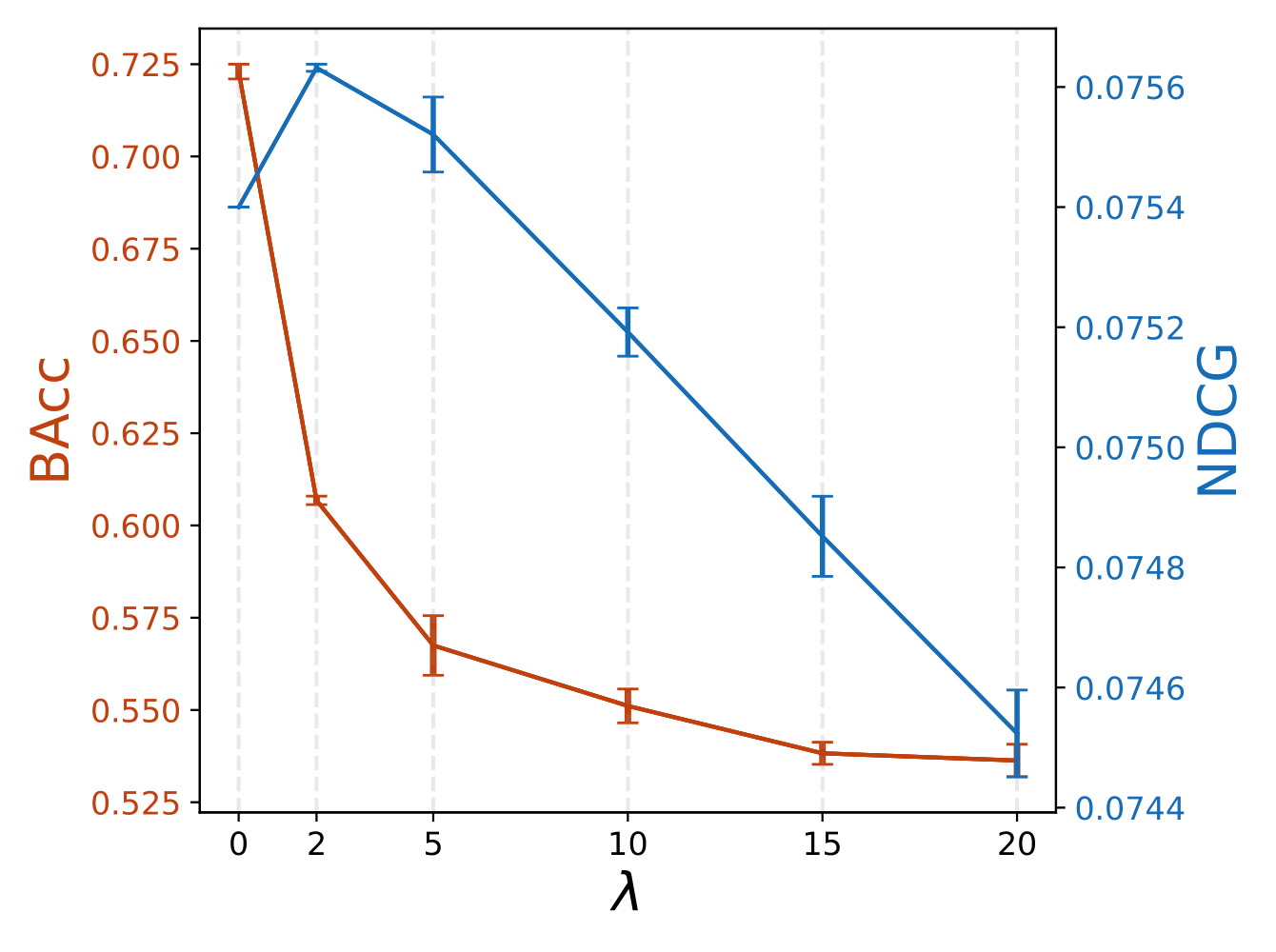
Abstract
Recommender Systems (RSs) may inadvertently perpetuate biases based on protected attributes like gender, religion, or ethnicity. Left unaddressed, these biases can lead to unfair system behavior and privacy concerns. Interpretable RS models provide a promising avenue for understanding and mitigating such biases. In this work, we propose a novel approach to debias interpretable RS models by introducing user-specific scaling weights to the interpretable user representations of prototype-based RSs. This reduces the influence of the protected attributes on the RS’s prediction while preserving recommendation utility. By decoupling the scaling weights from the original representations, users can control the degree of invariance of recommendations to their protected characteristics. Moreover, by defining distinct sets of weights for each attribute, the user can further specify which attributes the recommendations should be agnostic to. We apply our method to ProtoMF, a state-of-the-art prototype-based RS model that models users by their similarities to prototypes. We employ two debiasing strategies to learn the scaling weights and conduct experiments on ML-1M and LFM2B-DB datasets aiming at making the user representations agnostic to age and gender. The results show that our approach effectively reduces the influence of the protected attributes on the representations on both datasets, showcasing flexibility in bias mitigation, while only marginally affecting recommendation quality. Finally, we assess the effects of the debiasing weights and provide qualitative evidence, particularly focusing on movie recommendations, of genre patterns identified by ProtoMF that correlate with specific genders.
Citation
Alessandro B.
Melchiorre,
Shahed
Masoudian,
Deepak
Kumar,
Markus
Schedl
Modular Debiasing of Latent User Representations in Prototype-based Recommender Systems
Proceedings of the European Conference on Machine Learning and Principles and Practice of Knowledge Discovery in Databases (ECML PKDD 2024),
56--72, 2024.
Best Student Paper Award
BibTeX
@inproceedings{Melchiorre2024ModDebias, title = {Modular Debiasing of Latent User Representations in Prototype-based Recommender Systems}, author = {Melchiorre, Alessandro B. and Masoudian, Shahed and Kumar, Deepak and Schedl, Markus}, booktitle = {Proceedings of the European Conference on Machine Learning and Principles and Practice of Knowledge Discovery in Databases (ECML PKDD 2024)}, publisher = {Springer}, location = {Vilnius, Lithuania}, pages = {56--72}, month = {September}, year = {2024} }