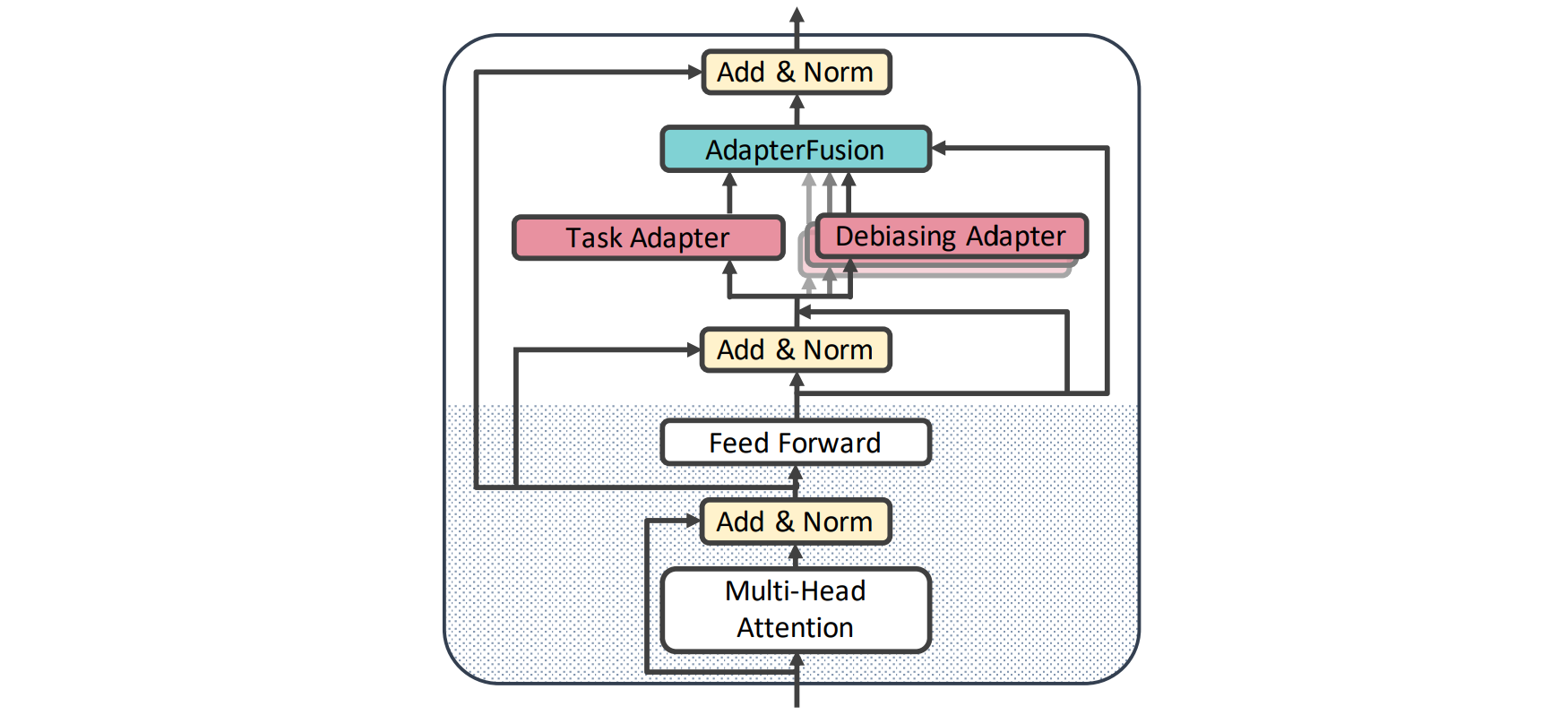
Abstract
Large pre-trained language models contain societal biases and carry along these biases to downstream tasks. Current in-processing bias mitigation approaches (like adversarial training) impose debiasing by updating a model’s parameters, effectively transferring the model to a new, irreversible debiased state. In this work, we propose a novel approach to develop stand-alone debiasing functionalities separate from the model, which can be integrated into the model on-demand, while keeping the core model untouched. Drawing from the concept of AdapterFusion in multi-task learning, we introduce DAM (Debiasing with Adapter Modules) – a debiasing approach to first encapsulate arbitrary bias mitigation functionalities into separate adapters, and then add them to the model on-demand in order to deliver fairness qualities. We conduct a large set of experiments on three classification tasks with gender, race, and age as protected attributes. Our results show that DAM improves or maintains the effectiveness of bias mitigation, avoids catastrophic forgetting in a multi-attribute scenario, and maintains on-par task performance, while granting parameter-efficiency and easy switching between the original and debiased models.
Citation
Deepak
Kumar,
Oleg
Lesota,
George Zerveas,
Daniel Cohen,
Carsten Eickoff,
Markus
Schedl,
Navid
Rekab-saz
Parameter-efficient Modularised Bias Mitigation via AdapterFusion
Proceedings of the 17th Conference of the European Chapter of the Association for Computational Linguistics,
2738–2751, 2023.
BibTeX
@inproceedings{Kumar2023DAM_EACL_2023, title = {Parameter-efficient Modularised Bias Mitigation via AdapterFusion}, author = {Kumar, Deepak and Lesota, Oleg and Zerveas, George and Cohen, Daniel and Eickoff, Carsten and Schedl, Markus and Rekab-saz, Navid}, booktitle = {Proceedings of the 17th Conference of the European Chapter of the Association for Computational Linguistics}, publisher = {Association for Computational Linguistics}, address = {Dubrovnik, Croatia}, url = {https://aclanthology.org/2023.eacl-main.201}, pages = {2738–2751}, month = {May}, year = {2023} }