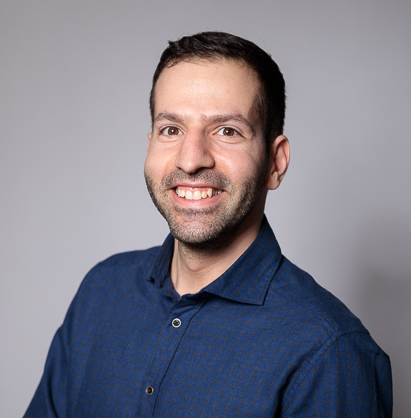
I am an assistant professor at Johannes Kepler University - JKU. Prior to it, I was a post doctoral researcher at Idiap Research Institute (affiliated with EPFL), and a PhD candidate at TU Wien. I explore deep learning methods in natural language processing and information retrieval, with a focus on fairness and algorithmic bias mitigation.
Contact
Univ. Ass., Dr. Navid Rekab-saz
Assistant Professor
Publications
Peer-Reviewed Journal and Conference Papers
Shahed Masoudian,
Koutini Khaled,
Markus Schedl,
Widmer Gerhard,
Navid Rekab-saz
Domain Information Control at Inference Time for Acoustic Scene Classification
31st European Signal Processing Conference, (EUSIPCO) 2023, Helsinki,Finland, September 4-8, 2023, 2023
Oleg Lesota,
Stefan Brandl,
Matthias Wenzel,
Alessandro B. Melchiorre,
Elisabeth Lex,
Navid Rekab-saz,
Markus Schedl
Exploring Cross-group Discrepancies in Calibrated Popularity for Accuracy/Fairness Trade-off Optimization
Proceedings of the 2nd Workshop on Multi-Objective Recommender Systems co-located with 16th ACM Conference on Recommender Systems (RecSys 2022), Seattle, WA, USA, 18th-23rd September, 2022
Oleg Lesota,
Emilia Parada-Cabaleiro,
Stefan Brandl,
Elisabeth Lex,
Navid Rekab-saz,
Markus Schedl
Traces of Globalization in Online Music Consumption Patterns and Results of Recommendation Algorithms
Proceedings of the 23rd International Society for Music Information Retrieval Conference, ISMIR 2022, Bengaluru, India, December 4-8, 2022